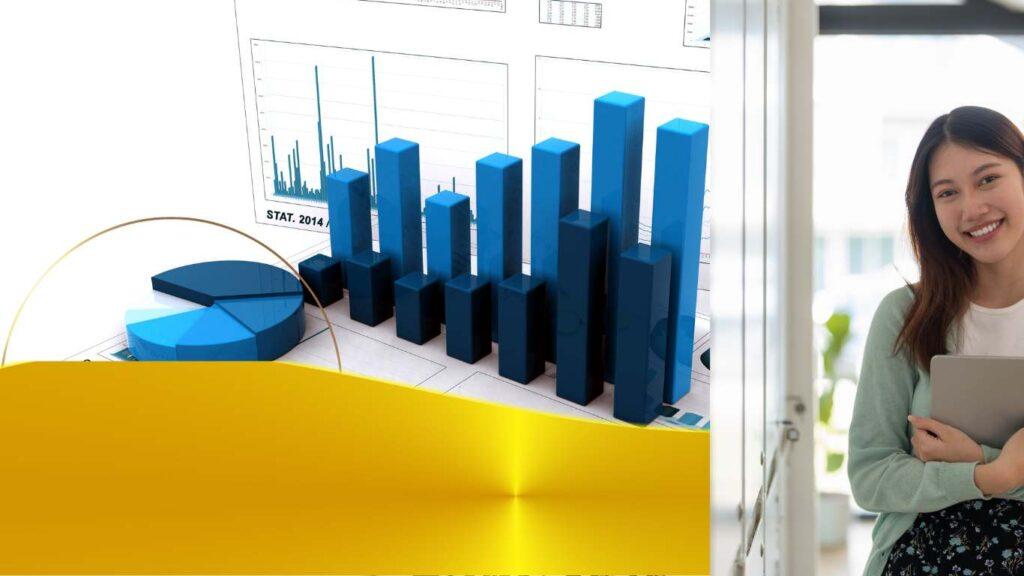
6 Steps To Follow When Analyzing Data Using SPSS
SPSS, which stands for Statistical Package for Social Sciences is a strong statistical tool mainly used for analysing the data in different ways. No matter if you’re working on a research project, using SPSS in data analysis for a dissertation, or a business report, or just exploring something on your own, SPSS can help you arrange, change, and visualize your data. It also helps you check your ideas and make decisions based on the data. In this article, you’ll discover six steps to use SPSS for understanding and analyzing data.
1. Step 1: Define your research questions/ hypotheses
Before you dive into SPSS data analysis, it’s important to know what you’re looking for and what you think you might discover. This means, you always start with defining research questions or research hypotheses before analyzing data using SPSS. Also, when defining the research questions, you should be clear on what you wish to achieve and whether your variables are measured in your data. In other words, some of the factors you should consider when formulating research questions include:
- Relevance to Your Field: Ensure that your research questions are closely related to your field of study and contribute to existing knowledge.
- Clarity and Precision: Craft clear and specific questions that avoid ambiguity.
- Feasibility: Make sure your questions are realistic and can be answered within the scope of your dissertation.
- Originality: Aim for questions that address gaps in existing literature or offer a unique perspective.
- Measurability: Ensure that your questions can be measured or assessed using appropriate methods and data.
Your hypothesis is like a guess about how things are connected or different. For instance, if you’re curious about how gender influences how well college students do in their studies, your research question could be: “Does gender make a difference in the academic performance of college students?” Your guess, or hypothesis, could be: “Female students do better in their studies than male students.”
2. Step 2: Prepare Your Data
Once you have your research question and hypothesis, you need to collect and prepare your data. Some of the tools you can use to collect data for SPSS data analysis include, survey questionnaires, existing databases, experiments and even observations. Once you have gathered data using your preferred data collection tool, you need to prepare and preprocess your data for analysis. That is, before analyzing data using SPSS, you need to perform some data transformation and organization to ensure it fits the suitable format that SPSS can read and process.
Some of the main steps you need to follow when preprocessing your data for spss data analysis include:
- Data Collection and Organization
- Collect all the relevant data for your study and ensure it is well-organized.
- Store data in a format that is compatible with SPSS (e.g., Excel, CSV).
- Data Cleaning
- Identify and handle missing data: Decide whether to remove cases with missing data, impute missing values, or use other appropriate techniques.
- Check for and correct any data entry errors or inconsistencies.
- Data Coding
- Assign meaningful labels to categorical variables (e.g., convert numeric codes to labels for gender: 1 = Male, 2 = Female).
- Ensure consistency in coding throughout the dataset.
- Data Transformation
- If necessary, perform transformations like log or square root to make data more normally distributed.
- Create new variables or recode existing ones to suit your analysis (e.g., calculating a mean score from multiple survey items).
- Outlier Handling
- Identify outliers using descriptive statistics or visualization techniques (e.g., box plots).
- Decide whether to remove, transform, or winsorize outliers, depending on the nature of your data and research objectives.
- Variable Selection
- Determine which variables are relevant to your research question and analysis. Remove irrelevant variables to simplify your dataset.
- Data Exploration
- Generate summary statistics and explore the distribution of your variables using SPSS descriptive statistics and visualizations (e.g., histograms, scatterplots).
Once you’ve completed these preprocessing steps, your data should be well-prepared for analysis in SPSS or any other statistical software, and you can proceed with your chosen statistical tests and research objectives.

Learn How to Analyze Data Using SPSS in 6 Steps
3. Step 3: Choose the Right Statistical Tests
There are various statistical tests available, each with its specific use cases. Depending on your research question and hypothesis, you need to select the most suitable statistical test that will help you answer your question and test your hypothesis.
SPSS offers a wide range of analysis methods, such as descriptive statistics, inferential statistics, correlation, regression, ANOVA, chi-square, t-test, and more. Remember that statistical tests highly rely on the level of measurements. Therefore, you need to be well-versed with the various levels of measurements.
Statistical Levels of Measurement
In statistics, there are four levels of measurement, which represent the different ways in which data can be categorized and analyzed. These levels are hierarchical, with each level including all the characteristics of the levels below it. The four levels of measurement are:
- Nominal Level (Categorical):
- Nominal data represents categories or labels for different groups or items.
- Data at this level cannot be quantitatively compared or ordered.
- Examples include gender (male, female), colors, types of animals, and yes/no responses.
- Statistical operations: Mode (most frequent category), frequency counts.
- Ordinal Level (Ordered Categorical):
- Ordinal data includes categories with a specific order or ranking.
- While you can rank and order the categories, the intervals between them are not equal or meaningful.
- Examples include education levels (e.g., high school, bachelor’s, master’s, Ph.D.), Likert scale responses (e.g., strongly disagree, disagree, neutral, agree, strongly agree).
- Statistical operations: Mode, median, percentiles, non-parametric tests.
- Interval Level:
- Interval data has equal intervals between values, but it lacks a true zero point.
- You can perform arithmetic operations like addition and subtraction on interval data, but multiplication and division do not make sense in the absence of a meaningful zero.
- Examples include temperature in Celsius or Fahrenheit and IQ scores.
- Statistical operations: Mode, median, mean, standard deviation, parametric tests (e.g., t-tests, ANOVA).
- Ratio Level:
- Ratio data has equal intervals between values and a true zero point.
- With a true zero, you can perform all arithmetic operations, including multiplication and division.
- Examples include height, weight, age, income, and counts (e.g., number of books).
- Statistical operations: Mode, median, mean, standard deviation, parametric tests, and ratio-based measures (e.g., income-to-debt ratio).
The level of measurement determines the types of statistical analysis that can be applied to the data. Typically, as you move up the hierarchy from nominal to ratio, you gain more analytical capabilities and precision. However, it’s important to choose the appropriate level of measurement for your data to ensure meaningful and accurate analysis. Using an inappropriate level of measurement can lead to incorrect conclusions and misinterpretation of results in statistical analysis.
Furthermore, you need to ensure that the assumptions for each statistical tests are met before proceeding with your analysis. For example, if you want to compare the means of two groups, you can use a t-test, but you need to check if your data is normally distributed and if the groups have equal variances. You will also need to understand the difference between parametric and non-parametric tests and when to use them.
4. Step 4: Analyzing Data Using SPSS
Once you’ve selected the most suitable statistical test, the next step is to conduct data analysis using SPSS and check your output. You can use the menus, the dialog boxes, or the syntax editor to run your analysis in SPSS. You can also customize your output options, such as tables, charts, graphs, or reports. You need to examine your output carefully and look for any errors, warnings, or messages that might indicate a problem with your data or analysis. You also need to interpret your output and look for the relevant statistics, such as means, standard deviations, p-values, coefficients, or effect sizes.
5. Step 5: Report your results and findings
Once you’ve figured out what your data is trying to tell you, it’s time to share your findings in a clear and simple way. You’ll want to follow the referencing guidelines such as APA, MLA, or Chicago style, to make it all look nice and organized.
You can share your results by writing about them, making tables or charts, and using the right words. Make sure to connect what you found to your original questions and hypothesis. For example, you might say something like this: The results of the t-test showed that female students had significantly higher academic performance than male students (M = 85.6, SD = 9.8; M = 79.4, SD = 10.2; t(98) = 3.12, p < 0.01). This means that the proposed research hypothesis has been proven thus confirming the hypothesis.
6. Step 6: Evaluate your analysis and interpretation
The last step involves carefully looking at your analysis and interpretation while thinking about their strengths and weaknesses. You should consider whether your analysis and interpretation are trustworthy and applicable to a broader context. It’s also essential to be aware of any potential biases, mistakes, or uncertainties that could affect your work and figure out how to minimize them. Additionally, you should suggest what your findings mean and any recommendations or future research ideas that might come from them.
For example, you could say: “Our study shows that gender plays a role in how well college students perform academically. However, we should keep in mind that our study had some limitations, like a small group of participants, only using one way to measure academic performance, and not taking into account other things that could affect academic success. So, it’s important to do more research to understand why there are differences between genders in how well students do in college.”
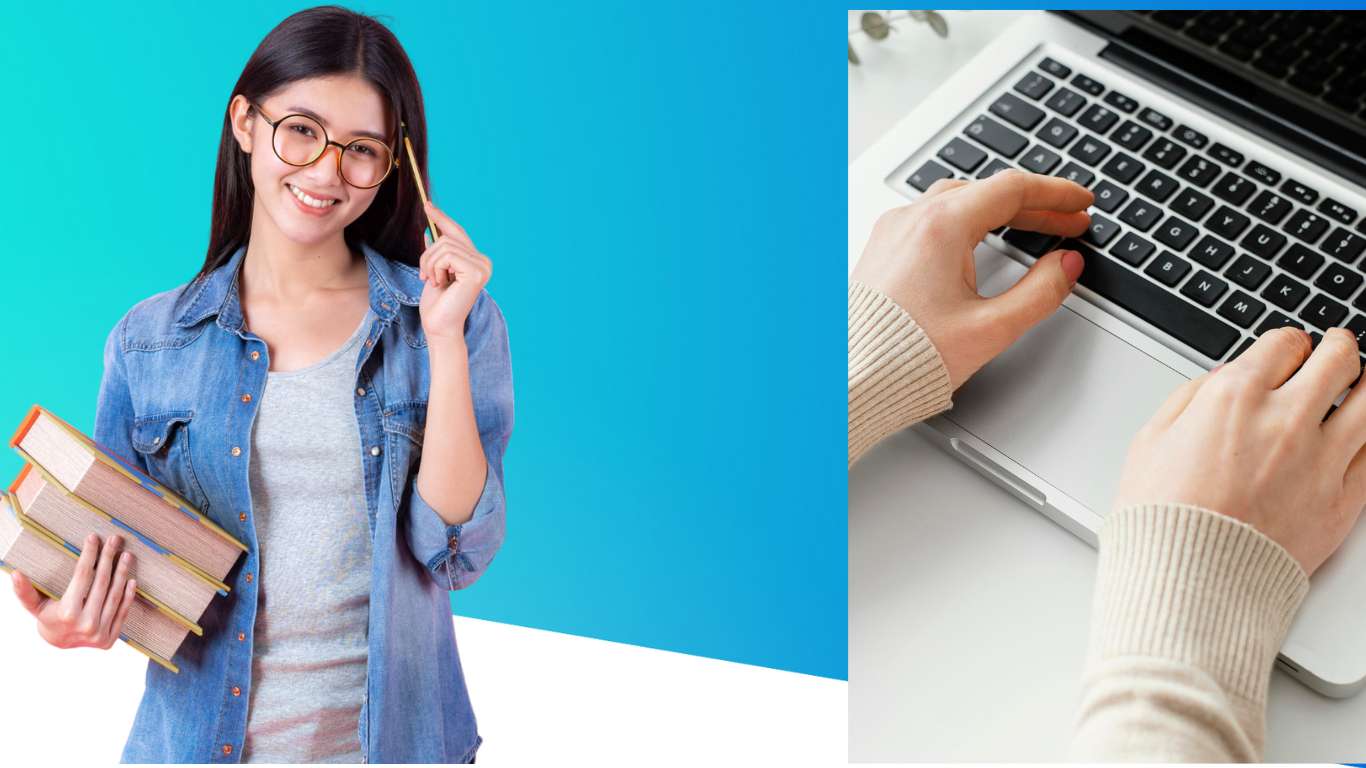
Still struggling with data analysis using SPSS? Worry no more! Online-SPSS have professional SPSS tutors to help you with any type of analysis using various statistical software.