Understanding the Difference Between Parametric and Non-Parametric Tests
Parametric and non-parametric tests are two fundamental concepts in statistical analysis. Understanding the difference between these two types of tests is crucial for researchers and analysts in selecting the appropriate statistical method for their data analysis. Parametric tests assume specific distributional properties of the data, while non-parametric tests make minimal assumptions about the underlying population distribution. This article aims to elucidate the differences between parametric and non-parametric tests. It starts by discussing parametric and non-parametric tests and their assumptions, then proceeds to highlight the key differences between these tests. Thus, the article provides a comprehensive understanding of parametric and non-parametric tests, their respective applications, and their implications in statistical inference.
Parametric Test: Definition and Assumptions
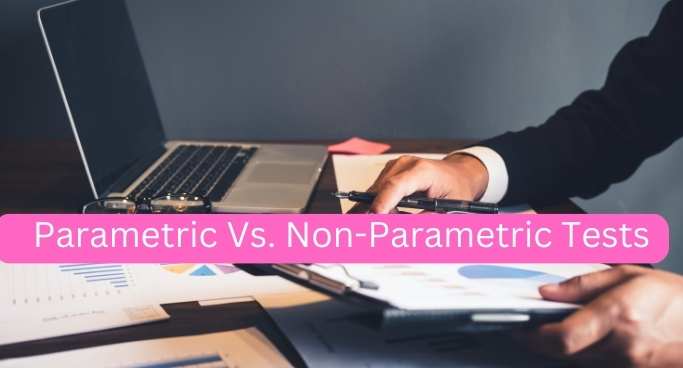
In the field of Statistics, a parametric test is a hypothesis test that aims to make inferences about the mean of the original population. This type of test is conducted based on parameters and assumes knowledge of the population distributions. The commonly used t-test utilizes the students’ t-statistic, which is applicable when specific conditions are met.
Parametric tests rely on the assumption of a normal distribution for the underlying variables. The mean value is considered, either known or estimated, while identifying the sample from the population. These tests are most effective when the variables of interest are measured on an interval scale.
Non-parametric Test: Definition and Characteristics
Contrary to parametric tests, non-parametric tests do not require any assumptions about the population distribution or distinct parameters. These tests are also hypothesis-based but do not rely on underlying assumptions. Instead, they focus on differences in medians and are often referred to as distribution-free tests.
Non-parametric tests are particularly suitable for variables determined on a nominal or ordinal level. They are commonly employed when dealing with non-metric independent variables. The central tendency is measured using the median value, and these tests offer flexibility by not relying on any specific probability distribution.
Key Differences Between Parametric and Non-parametric Tests
The key differences between parametric and non-parametric tests can be summarized as follows:
- Assumptions: Parametric tests require assumptions about the data distribution, while non-parametric tests do not have such assumptions.
- Central Tendency Value: Parametric tests use the mean value to measure central tendency, whereas non-parametric tests use the median value.
- Correlation: Parametric tests employ Pearson correlation, while non-parametric tests use Spearman correlation.
- Probabilistic Distribution: Parametric tests assume a normal distribution, while non-parametric tests can be applied to arbitrary distributions.
- Population Knowledge: Parametric tests require knowledge about the population, whereas non-parametric tests do not have this requirement.
- Applicability: Parametric tests are suitable for interval data, while non-parametric tests are used for nominal data.
- Examples: Parametric tests include z-test and t-test, while non-parametric tests include Kruskal-Wallis and Mann-Whitney.
Examples of parametric tests and Their Non-Parametric Test Equivalents
Parametric tests | Non-parametric tests Equivalent |
Paired t-test/dependent t-test | Wilcoxon signed-rank test |
Pearson’s Correlation | Spearman’s Correlation |
Independent sample t-test | Mann-Whitney U-test |
One-Way ANOVA | Kruskall-wallis test |
Two-Way ANOVA | Friedman test |
Final Take Away
- The key difference between parametric and non-parametric tests lies in their fundamental principles and statistical approaches. While parametric tests rely on statistical distributions within the data, nonparametric tests do not depend on any specific distribution.