WhatsApp: +1-(424)-285-0253
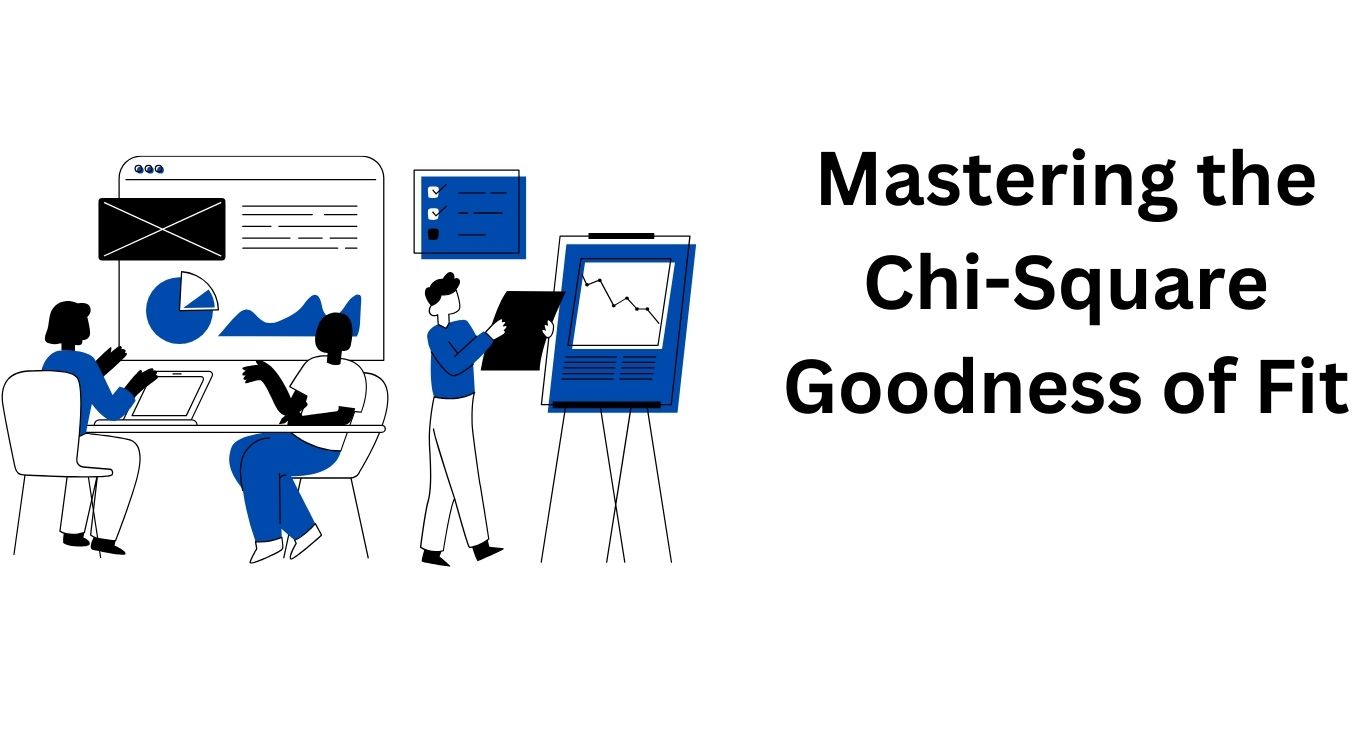
The Chi-square goodness of fit test is a non-parametric statistical test used to determine the extent to which observed values deviate from expected values. This test enables the comparison of an observed sample distribution with an expected probability distribution, assessing how well theoretical distributions such as normal, binomial, or Poisson fit the empirical data. By dividing sample data into intervals and comparing the number of data points falling into each interval with the expected values, one can ascertain the goodness of fit.
Procedure for Conducting the Chi-Square Goodness of Fit Test:
Formulating the Hypotheses
Null Hypothesis: The null hypothesis for the Chi-Square goodness of fit test posits that there is no significant difference between the observed and expected values.
Alternative Hypothesis: Conversely, the alternative hypothesis assumes a significant difference between the observed and expected values.
Calculating the Chi-Square Statistic
The Chi-Square goodness of fit test computes the value of the Chi-Square statistic using the following formula:
Chi-Square = Σ [(O – E)^2 / E]
Where: O represents the observed value,
E represents the expected value.
Determining Degrees of Freedom
The degrees of freedom for the Chi-Square goodness of fit test depend on the distribution of the sample.
“Unlock the potential of Chi-Square tests with Online-SPSS.com‘s expert tutors. Gain the confidence and skills you need for accurate statistical analysis.”
Interpretation of Chi-square goodness of Fit
The process of hypothesis testing in the Chi-Square goodness of fit test is similar to other tests such as t-tests and ANOVA. The calculated Chi-Square statistic is compared against the critical value from the Chi-Square distribution table.
If the calculated value exceeds the critical value, the null hypothesis is rejected, indicating a significant difference between the observed and expected frequencies.
Conversely, if the calculated value is lower than the critical value, we fail to reject the null hypothesis, implying no significant difference between the observed and expected values.
By employing the Chi-Square goodness of fit test, researchers and statisticians can evaluate the conformity of observed data to expected distributions. This test provides valuable insights into the statistical significance of deviations, facilitating informed conclusions about the fit between empirical and theoretical distributions.
[order_button_dark]
GET SPSS HELP FROM ONLINE SPSS
There are various statistical software available today. Among these statistical software, SPSS is the most widely used, especially by university students when writing their thesis and dissertation papers. If you’re an Undergraduate student, a Master’s student, a PhD student, you must have encountered data analysis using SPSS and if not yet, then you will before the end of your studies.
Despite its significance in most fields, running data analysis using SPSS is often challenging. This is where Online-SPSS comes in. If you’re a student in need of help with SPSS Analysis, then we got you covered. Our professional team of data analysis experts work tirelessly to help you offer you with SPSS data analysis assistance you may need.
Whether you are looking for spss help for dissertation, or spss assignment help, our SPSS tutors will provide you with step-by-step solutions that guarantee an A+ grade. We understand that performing statistical analysis using SPSS by yourself is always cheaper. However, it can also be time-consuming, which can even lead to poor grades.
If you’re not proficient in SPSS, then performing statistical analysis using SPSS is not a good idea. It can take days just to figure out how to do some of the easier things in SPSS. So paying someone to do your SPSS will save you a huge amount of time and make your life a lot easier.
The procedure of getting SPSS help service at Online SPSS is very simple. You only need to follow the following simple steps;
1. Click the ORDER NOW button
2. Make Secure Payment
3. Get the Solution
Our main focus is to provide quick, reliable, and professional data analysis services using SPSS and other statistical software.